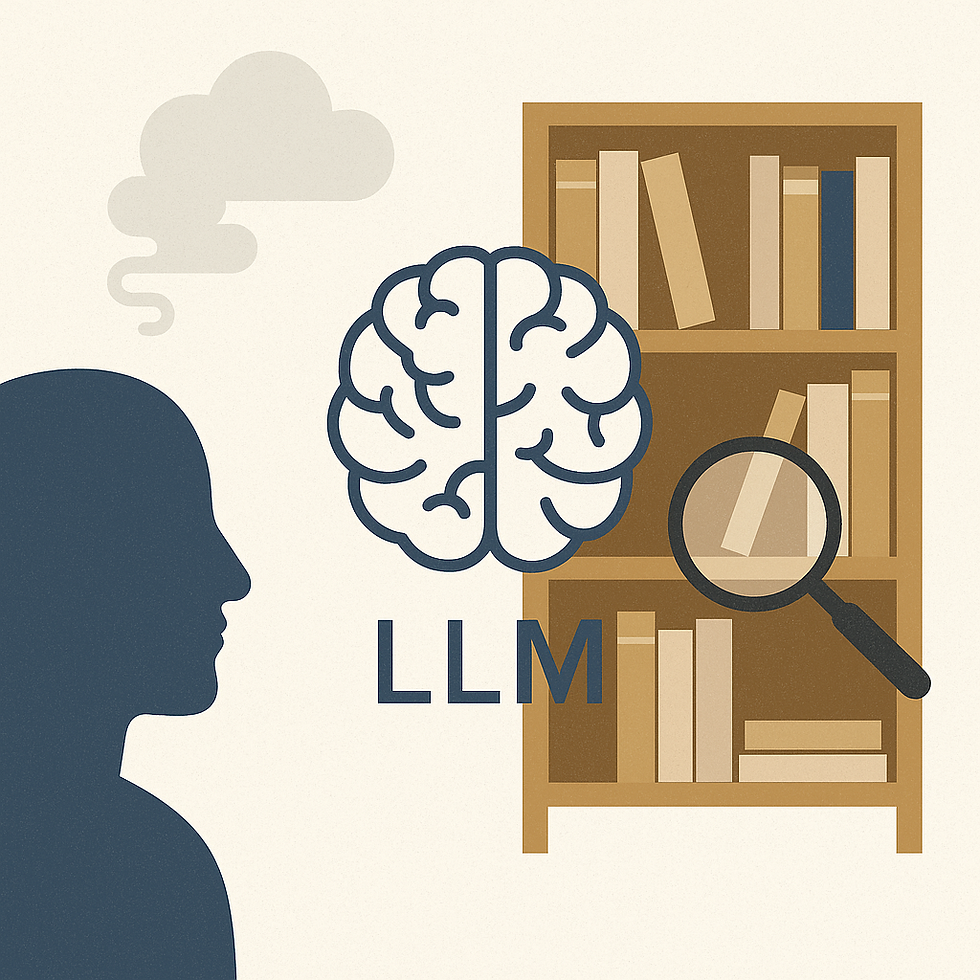
AI & UXR, CHAT GPT, LLM
RAGSs Against Hallucinations – Well Thought Out, but Not Good Enough?
Why retrieval-augmented generation is not a cure-all for reliable AI responses
2
MIN
May 20, 2025
In the world of artificial intelligence (AI), hallucinations – i.e. the invention of plausible-sounding but incorrect information – are a well-known problem with large language models (LLMs). Retrieval-augmented generation (RAG) has been presented as a solution to minimise this problem by incorporating external, reliable data sources into the response generation process. But is RAG really the silver bullet for hallucinations?
What is RAG anyway – and why is it relevant?
RAG combines an LLM with an information retrieval system. Instead of relying solely on the knowledge stored in the model, the system searches external databases for relevant information and uses it to generate more accurate responses. For UX experts, this means that AI can access current and specific information, which is particularly advantageous in dynamic application areas.
What RAGs do really well
Integration of current information: RAG enables access to current data that has been created after the LLM has been trained.
Reduction of hallucinations in factual questions: Access to reliable sources reduces false claims.
Transparency through source references: Answers can be provided with direct references to the sources used, which increases traceability.
Application in companies: RAG can help provide specific information efficiently, especially in internal knowledge systems.
But: Why RAGs are often overrated
Despite the advantages, there are also challenges:
Quality of the retrieved data: If the system retrieves irrelevant or outdated information, this can lead to incorrect answers.
Misinterpretation by the LLM: Even with correct data, the model may misunderstand or weigh it incorrectly.
Technical effort: Implementing and maintaining an effective RAG system requires significant resources.
Real-world example: A study by Stanford University showed that specialised legal AI tools that use RAG hallucinate in 17–33% of cases. VentureBeat+3reglab.stanford.edu+3Legal Dive+3
Typical misconceptions about RAGs
"With RAG, AI never hallucinates.": This is a fallacy. RAG reduces hallucinations, but does not eliminate them completely.
"External data = true answers": The quality and relevance of the data are crucial.
"RAG = better LLMs": Without careful implementation and monitoring, RAG can actually worsen performance.
What a good RAG system must deliver
High-quality data sources: The data used must be current, relevant and reliable.
Effective search algorithms: The system must be able to find the most relevant information efficiently. Legal Tech Insights from Prevail
Contextual adaptation: The LLM should correctly interpret the retrieved data and place it in the right context.
Continuous monitoring: Regular reviews and adjustments are necessary to ensure quality.
Conclusion: RAG – a building block, not a silver bullet
RAG offers promising approaches for improving the response accuracy of LLMs, but it is not a panacea. For UX professionals, this means that RAG can be a valuable tool, but it should be used with caution and in combination with other methods to develop truly reliable and user-centred AI applications.
RELATED ARTICLES YOU MIGHT ENJOY
AUTHOR
Tara Bosenick
Tara has been active as a UX specialist since 1999 and has helped to establish and shape the industry in Germany on the agency side. She specialises in the development of new UX methods, the quantification of UX and the introduction of UX in companies.
At the same time, she has always been interested in developing a corporate culture in her companies that is as ‘cool’ as possible, in which fun, performance, team spirit and customer success are interlinked. She has therefore been supporting managers and companies on the path to more New Work / agility and a better employee experience for several years.
She is one of the leading voices in the UX, CX and Employee Experience industry.
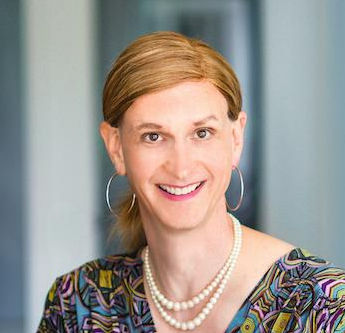