
AI & UXR, CHAT GPT
The Environmental Impact of AI – Why Sustainability Also Matters for Digital Innovation
3
MIN
Mar 25, 2025
Why is the environmental impact of AI important?
Artificial intelligence is changing our world. It simplifies processes, revolutionizes industries and offers numerous possible applications – from analysing medical data to automating business processes. But as AI's benefits grow, so does its environmental footprint. Many see AI as an invisible force that is integrated into systems ‘just like that’. But in fact, it is backed by an energy-intensive infrastructure with significant environmental impacts. Today, AI applications already consume as much energy as a large city. The central question is: how much environmental pollution can we afford, and do we want to afford?
The biggest negative environmental impacts of AI
Energy consumption and carbon footprint of large AI models
AI is an energy guzzler. Particularly large models such as GPT-4 from OpenAI require enormous amounts of electricity for their training and application. A study by the Massachusetts Institute of Technology (MIT) shows that training an AI model generates as much CO₂ as five cars over their entire lifespan. Even more worrying: the global energy consumption of AI could be equivalent to the annual electricity demand of a large city like New York. (Source: https://taz.de/Oekologischer-Fussabdruck-von-KI/!5946576/)
Water consumption for cooling data centres
Data centres that run AI models not only require electricity, but also huge amounts of water for cooling. In hot climates, this exacerbates water scarcity. For example, a data centre in West Des Moines, Iowa, used around 6% of the city's total water supply in July 2022. This is increasingly becoming a problem in water-scarce regions. (Source: https://www.nature.com/articles/d41586-024-00478-x)
Material consumption and electronic waste due to specialised hardware
AI relies on specialised hardware such as GPUs and TPUs, which contain rare raw materials such as cobalt and silicon. The mining of these materials is not only ecologically questionable, but also socially problematic. In addition, rapid technological progress means that hardware is quickly replaced – with increasing amounts of electronic waste. (Source: https://www.t-online.de/digital/aktuelles/id_100416212/chatgpt-umweltbilanz-wie-viel-energie-die-ki-verbraucht.html)
Storage requirements for ever-larger amounts of data
Machine learning and AI require enormous amounts of data to be stored. Studies show that the storage requirements of AI systems are growing by about 20% annually. More data means more energy consumption for storage and processing. (Source: https://journalofbigdata.springeropen.com/articles/10.1186/s40537-024-00920-x)
Inequality in access to AI
The high energy requirements of AI make it a technology that primarily industrialized nations can afford. This widens the digital divide: while wealthy countries benefit from AI, regions with fewer resources are often excluded. (Source: https://www.dw.com/de/wie-k%C3%BCnstliche-intelligenz-der-umwelt-schadet/a-66305844)
Solutions: What can be done?
‘Green AI’ and more efficient models
Researchers are working on AI models with reduced energy consumption. One example is MIT's ‘Liquid Neural Networks’, which are more flexible and economical than conventional networks. (Source: https://www.wired.com/story/liquid-ai-redesigning-neural-network/)
Data centres powered by renewable energy
Google wants to be carbon neutral by 2030 and is already using solar and wind energy in some of its data centres. Other tech companies are also optimising their energy sources. (Source: https://www.welt.de/wirtschaft/webwelt/article252321534/Googles-grosses-Oeko-Ziel-wackelt-aus-zwei-Gruenden.html)
Improve code efficiency
Cleaner, more resource-efficient code saves energy. Developers can significantly reduce power consumption through optimised programming. Tools are available to identify inefficient code. (Source: https://www.hosteurope.de/blog/ki-code-generatoren-wie-ki-webentwicklern-hilft-code-effizienter-zu-schreiben-und-fehler-schneller-zu-finden/)
Location optimisation of data centres
Cooler regions reduce the energy and water requirements for data centres. Iceland, for example, uses geothermal energy and the cold climate for particularly efficient server farms. (Source: https://reset.org/wie-wird-der-energiefresser-ki-nachhaltiger/)
Political incentives for sustainable AI
Governments can provide incentives for sustainable AI research. Germany is funding projects for the resource-efficient use of AI. (Source: https://www.bmuv.de/pressemitteilung/grosser-schritt-fuer-ki-und-umwelt-bmuv-zeigt-erste-ergebnisse-der-green-ai-hub-pilotprojekte)
Sustainable use of AI in everyday work: practical tips
Use AI tools efficiently: Avoid unnecessary requests.
Raise awareness: Every AI request costs energy, especially computationally intensive tasks.
Favour smaller, specialised models: A less energy-intensive solution is often sufficient.
Use long-lasting hardware: Modular, upgradable devices avoid unnecessary electronic waste.
Use green AI tools: Some AI applications rely on energy-efficient architecture.
With these measures, we can use AI more responsibly. Or just use our brains for a change. Well.
RELATED ARTICLES YOU MIGHT ENJOY
AUTHOR
Tara Bosenick
Tara has been active as a UX specialist since 1999 and has helped to establish and shape the industry in Germany on the agency side. She specialises in the development of new UX methods, the quantification of UX and the introduction of UX in companies.
At the same time, she has always been interested in developing a corporate culture in her companies that is as ‘cool’ as possible, in which fun, performance, team spirit and customer success are interlinked. She has therefore been supporting managers and companies on the path to more New Work / agility and a better employee experience for several years.
She is one of the leading voices in the UX, CX and Employee Experience industry.
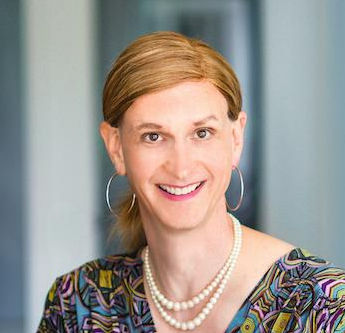